Unlocking the Future of Agriculture with Machine Learning Datasets
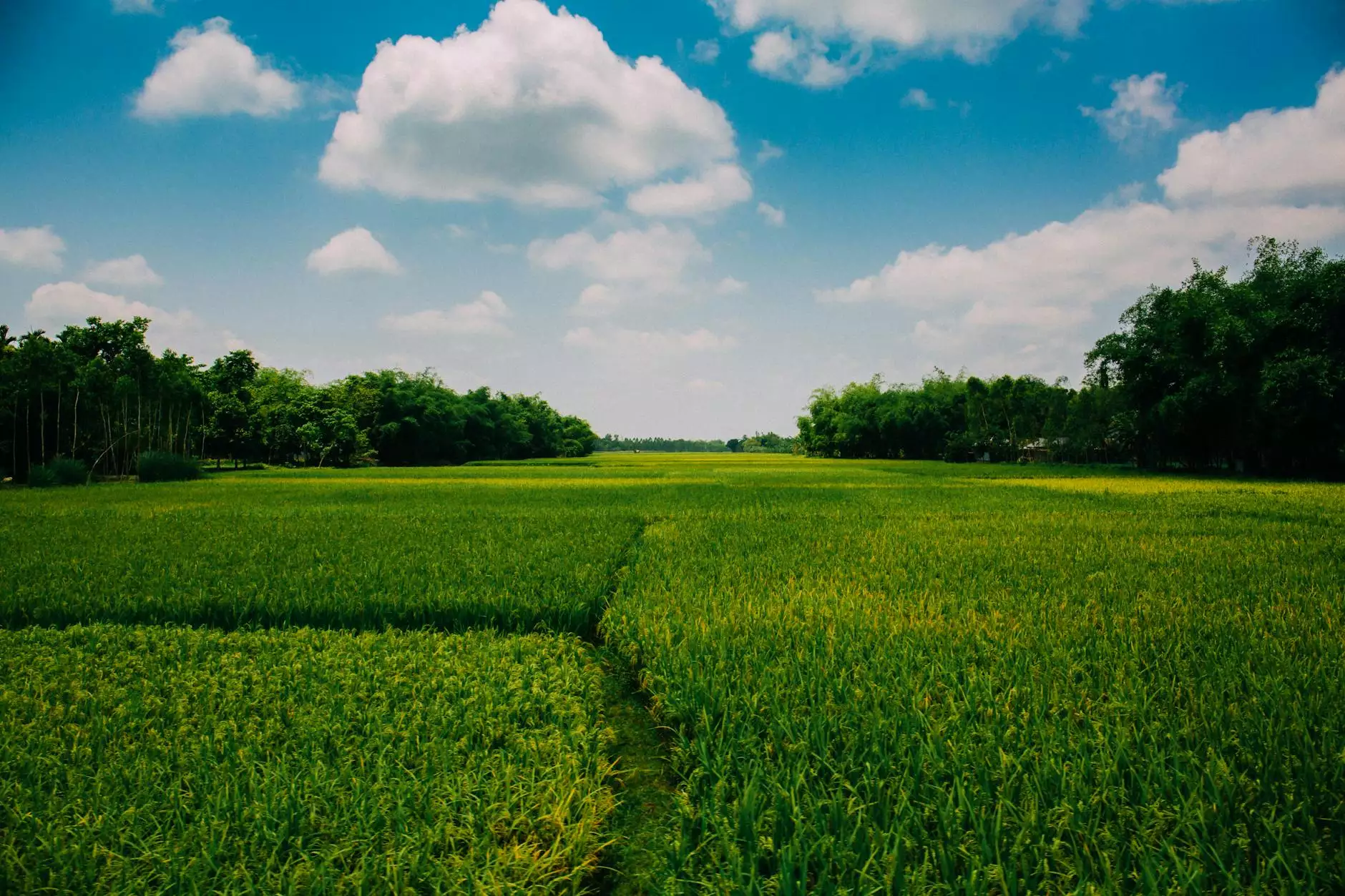
The agricultural sector is witnessing a transformative shift driven by technological advancements. Among these, machine learning and data science stand out as powerful tools that can revolutionize farming practices and enhance productivity. In this article, we will delve into the significance of utilizing an agriculture dataset for machine learning, how it can empower farmers and businesses alike, and the expansive potential it holds for the future of agriculture.
Understanding Agriculture Datasets
To appreciate the role of machine learning in agriculture, we first need to understand what agriculture datasets entail. These datasets are collections of structured information that pertain to various agricultural practices, crop yields, soil types, weather data, pest infestations, and much more. Below, we explore the types of datasets that can be harnessed:
- Crop Yield Data: Information regarding the yield from various crops over multiple seasons.
- Soil Quality Data: Details about soil composition, pH levels, and nutrient content.
- Weather Patterns: Historical and predictive meteorological data that can affect farming.
- Pest and Disease Reports: Records of pest outbreaks and crop diseases that challenge farmers.
- Market Prices: Trends and fluctuations in agricultural commodity prices.
The Importance of Data in Modern Agriculture
Whether it’s a large-scale farming operation or a small family-run farm, the use of data has become increasingly vital to make informed decisions. Here's why harnessing data is crucial:
- Improved Decision Making: Access to accurate data helps farmers make informed decisions on planting, harvesting, and marketing.
- Resource Management: Efficient use of water, fertilizers, and pesticides based on data-driven insights reduces waste and maximizes profits.
- Yield Maximization: Analyzing yield data to identify the best practices that lead to higher crop production.
- Risk Mitigation: Predictive analytics can alert farmers to potential risks like drought or pest outbreaks, enabling proactive measures.
- Sustainability Practices: Utilizing data to adopt ecological farming methods that protect our planet's resources.
The Role of Machine Learning in Agriculture
With the advent of machine learning, the agricultural sector has a powerful ally in data analysis. Machine learning algorithms can process vast amounts of agricultural data, uncover patterns, and provide actionable insights that were previously out of reach. Here are some applications where machine learning shines:
1. Predictive Analytics
Machine learning models can analyze historical data to predict future crop yields, pest invasions, and weather patterns. This predictive capability is critical for planning and optimizing resources.
2. Precision Farming
With data collected from sensors and drones, machine learning can help farmers practice precision agriculture. This approach ensures that crops receive the right amount of nutrients and water at the right time.
3. Automated Decision Making
Combining machine learning algorithms with real-time data can lead to automated systems that actively manage irrigation, fertilization, and other farming practices without human intervention.
4. Disease Detection
Machine learning can be utilized in image recognition to identify plant diseases early, enabling quick action to mitigate losses.
5. Supply Chain Optimization
Analyzing market data and trends helps farmers and distributors streamline operations and maximize profits while minimizing waste.
Leveraging Agriculture Datasets for Machine Learning
For machine learning to be effective in agriculture, it is essential to have access to high-quality datasets. Developing models that yield accurate predictions and insights relies heavily on the quality, diversity, and richness of the data being fed into them.
Where to Find Agriculture Datasets for Machine Learning
Here are some fantastic resources where you can access agriculture datasets for machine learning:
- Kaggle: A popular platform for datasets, where users can find comprehensive datasets related to agriculture.
- Government Open Data Portals: Many governments provide access to agricultural data through their open data initiatives.
- Research Institutions: Universities often conduct agricultural research and publish datasets that are publicly available.
- AgriTech Companies: Numerous tech companies focus on agriculture and offer data solutions and datasets, either free or for purchase.
Challenges in Utilizing Agriculture Datasets
Despite their potential, several challenges exist when utilizing agriculture datasets for machine learning:
1. Data Quality and Consistency
The effectiveness of machine learning models is heavily influenced by the quality of the input data. Inconsistent, outdated, or inaccurate data can lead to erroneous predictions and poor decisions.
2. Integration of Data Sources
Data in agriculture can come from various sources: sensors, satellites, historical records, and more. Integrating these disparate sources into a cohesive dataset can be complex and time-consuming.
3. Skill Gap
Farmers and agricultural workers may lack the technical expertise required to analyze data or implement machine learning solutions, creating a gap between potential and actual usage.
4. Infrastructure Limitations
Rural areas may have limited access to the infrastructure required for data-based farming, including internet connectivity and advanced technological equipment.
Future Prospects of Agriculture Datasets and Machine Learning
The future of agriculture lies at the intersection of technology and tradition, with machine learning datasets leading the way towards a more efficient and sustainable agricultural model. Here are some promising trends and prospects:
1. Enhanced Artificial Intelligence Integration
As artificial intelligence continues to evolve, its integration with agricultural datasets will drive more sophisticated predictions and automate numerous farming processes.
2. Increased Adoption of IoT Devices
Internet of Things (IoT) devices will become increasingly commonplace, collecting data in real time to feed machine learning algorithms for better decision-making.
3. Sustainable Farming Practices
Utilizing machine learning can lead to more sustainable practices by optimizing resource allocation and minimizing the environmental impact of farming.
4. Global Collaborative Databases
International collaborations will likely lead to comprehensive databases that enhance data richness, allowing for more robust machine learning applications on a global scale.
Conclusion: The Path Ahead for Agriculture and Technology
In conclusion, the integration of agriculture datasets for machine learning is set to reshape the agricultural landscape significantly. By leveraging these datasets, farmers can make data-driven decisions that boost productivity, enhance sustainability, and mitigate risks. The rise of technology in agriculture heralds a new era, one where innovation meets tradition—ultimately paving the way for a sustainable, efficient future for all involved in the agricultural sector.
For businesses involved in Home Services, Keys & Locksmiths, understanding the implications of agricultural technology is essential for anticipating market trends and adapting to the evolving economic landscape. As the agriculture sector expands its reliance on data and machine learning, associated industries should also consider how these advancements can lead to interconnected opportunities.
Embracing technology is no longer optional in today’s agricultural environment; it is imperative to thrive and compete. As more data becomes available and machine learning techniques evolve, the ability to harness this information will differentiate successful operations from those that merely survive.